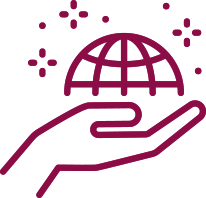
All About Data
This is not speculation. Every prediction is grounded in data from reliable sources.
Discover the ten definitive trends in artificial intelligence that will reshape the world this year. Get ahead of the game: download the report today.
Not everyone gets to see the future. But if you know where to look, you can. This definitive report distills down the thinking of some of the brightest minds in AI, helping you spot the ten big trends that will emerge in 2022, enabling your company to get one step ahead.
This is not speculation. Every prediction is grounded in data from reliable sources.
Glean insights from CEOs, strategists, research fellows, consultants, data scientists, and more.
Read about what’s coming and learn how others are already putting new technology to use.
Artificial intelligence surprises us at every step. Increasingly advanced technologies and exciting new projects pop up every year, and they never cease to amaze.
Even solutions that seemed like a vision of the distant future just a few years back are now becoming a reality. And businesses are waking up to AI’s growing potential, alongside the benefits that automation can deliver.
AI is now an integral part of many organizations. Developments in the field keep revealing new opportunities for businesses. But while AI has come so far, the question remains… what does 2022 hold in store?
I hope we’ll see even more surprising and world-changing solutions.
I firmly believe that people will begin to see the benefits of AI and use it for worthy causes that improve our lives. That’s why I believe Ethical AI is such an important topic today, and I hope it will be one of the most critical trends in 2022.
But I don’t want to give too much away at the top. Please dive into my full take on what will make the headlines in 2022 and enjoy insights from some of the greatest minds in AI.
I hope you enjoy the read!
Research specialists Gartner forecast that global artificial intelligence software revenue will reach $62.5 billion in 2022. That would be a 21.3% increase to 2021, which shouldn’t come as a surprise. More and more businesses are investing in AI to improve performance. And that has to be a good thing, right?
That said, one of the risk factors mentioned by Richard Kieffer in Managing Your AI-ML Journey for Success is the “Inability to integrate people, processes, and technology into a seamless business system.” The challenge is that most companies follow one pattern of implementing AI, but they don’t think about how it’ll affect their current workforce and systems.
That’s why 2022 will see an increase in AI-ML initiatives used to transform an organization’s core business processes, spanning both workflows and workforces.
I know of many projects where the “operation was a success, but the patient died.” In other words, the project delivered a functional technical solution, but the system failed to meet its business goals, or in some cases, the workforce rejected it.
In 2022 and beyond, I see an increased focus on leveraging AI-ML technologies to transform an organization’s core business processes to improve productivity, service, quality, and safety.
This shift will present several challenges to corporate leaders and AI-ML technology providers. The main one will understand the importance of human factors – i.e., integrating people, processes, and technology.
Edge Artificial Intelligence is the amalgamation of AI and computing. It runs ML algorithms directly on local devices, including smartphones, speakers, laptops, self-driven cars, even surveillance cameras. Edge AI doesn’t use cloud-based big data; instead, it relies on small data. Moreover, the core system doesn’t need to connect to others, meaning they can process data in real-time.
According to Research and Markets, the global Edge AI software market will reach over $8 billion by 2027.
Edge AI is becoming increasingly popular right now. It’s the next stage of development for IoT systems. With Edge AI, IoT devices are becoming more intelligent to deliver better experiences and results.
That means that with ML, edge devices are now able to make decisions. They can make predictions, process complex data and administer solutions. In essence, both edge and cloud computing are meant to do the same things – process data, run algorithms, etc.
You may have seen this one coming. After all, Mark Zuckerberg recently announced that Facebook was working on a metaverse: this virtual reality world based on VR technology. He then went one step further and changed Facebook’s name to Meta.
Now — while the general concept is still in its early phase, hundreds of companies are working on solutions designed for the metaverse. Which is why forecasts suggest the industry could be worth $800 billion by 2024.
Many big players are working on related products. Epic Games announced a $1 billion investment in its metaverse on April 13th — while Nvidia has designed its version of virtual reality called the NVIDIA Omniverse: a technology used by businesses to create real-world simulations of buildings, engineering, vehicles, manufacturing, media and entertainment, and game development, among other things.
Microsoft has also released Microsoft Mesh: a tool that uses mixed reality to connect people virtually via smartphones, tablets, VR headsets, PCs, HoloLenes, or any Mesh-enabled app.
One of the most significant new things announced in 2021 was, without any doubt, Meta and Metaverse. Keeping aside high hardware needs for this and the timing of their availability to the broad audience, they open the door to a wide variety of Deep Fake use-cases.
Deep Fake technology is computational consuming, but Expression Camera showed that it is possible to run it on modest hardware. Still, the potential is accurate, and I expect a wave of progress here or initiating it at least.
People often struggle to understand why an algorithm makes a particular recommendation, and that’s understandable. The output comes from a “black box,” which means you know the inputs, you get to see the result, but you don’t know what happened in between.
It can even be challenging for the inventors to explain why AI made a specific decision. But now, we have a solution: Explainable AI (XAI).
Explainable AI refers to a toolset that helps people understand and interpret the predictions made by an AI model. Which, in turn, helps us understand why a model makes a specific decision, spot potential errors, and improve overall performance.
Forecasts suggest the XAI market will grow six-fold between 2019 to 2030, exceeding $21 billion as companies look to:
In the context of the development of emerging technologies in general and artificial intelligence in particular, it is likely that we will see even more simplification and democratization.
We are already seeing the flourishing of no-code and low code platforms that allow the use of artificial intelligence and machine learning in various areas, domains, fields and sectors, such as education, logistics and transportation, or agriculture.
The growing societal need for Explainable AI will impact hiring AI behaviour forensic, privacy and customer trust specialists. They must reduce brand & reputation risk.
The role of the ML Forensic and Ethics Investigator is to ensure that all bias no longer exists within an ML system. Large corporations such as NASA, Google, and even Facebook say that they already have such individuals on their payroll.
GPT-3 is a natural language system invented by OpenAI. It can generate human-like text and perform tasks like translating, answering questions, suggest extemporaneous text (i.e., for chatbots), limiting language translation, even writing computer code.
Now, OpenAI has announced it’s working on GPT-4: a new version that’s supposed to be more potent than GPT-3. Details are still secret, but some believe that GPT-4 may contain up to 100 trillion parameters, making it 500x larger than the current version.
NLP is the main topic in AI today, and I can’t foresee any reason why this would change soon. GPT-3 and other similar models remain state-of-the-art in today’s AI.
That will continue in 2022, with slightly moving the direction of the progress from size to the technique. The new wave is already present on the horizon with DeepMind’s RETRO model. Expecting more and better models here.
We used to need massive datasets to build machine learning models. But thanks to new options like few-shot (FSL) and zero-shot learning (ZSL), that’s no longer the case.
Few-shot only needs a few training samples with supervised information — while zero-shot can class and predict new classes on data it has never seen before. ZSL is inspired by a human’s ability to recognize unknown classes using knowledge from known classes.
The level of similarity between the two datasets guides the model’s decision. The ultimate goal of this technique is to use limited data to train a model.
GPT-3 model developed in 2020 and the rise of its commercial use in 2021 showed that language models could be few-shot and even zero-shot-learners. However, GPT-3 was trained on an abundance of data with GPU power far outside the reach of any individual or a small organization.
In my opinion, in 2022, we will continue to focus on few-shot learning through large-scale pre-training and find better proxy tasks suitable for smaller and more efficient models. An example of this is prototypical models that can use both labelled and unlabeled data in their training.
We can also see the divergence from classic classification models to query-based models, leveraging the information in the class itself to further improve few-shot learning.
As we continue to see the democratization of AI, coupled with a growing demand for developers, there’s an increasing need for ‘citizen developers’ (by this, we mean people who can build applications using no-code or low-code platforms).
Citizen developers work in the business unit, harnessing their business skills to build IT solutions. This trend will only grow as we continue to see a lack of skilled programmers in the years ahead.
The so-called “citizen developers” or “non-technical programmers” are the most important link in the next cognitive stage of the digital transformation of organizations. Of course, they would not replace the systemic solutions offered by IT teams.
Still, they play a key role in prototyping specialized paths for effective collaboration with AI, which relieves stress in routines and unleashes individuals and teams’ creative potential.
The transformer in natural language processing (NLP) solves sequence-to-sequence tasks. It depends wholly on self-attention to compute its input and output representations without using sequence-aligned RNNs or convolutions.
When we talk about ‘multimodal’ transformers, we mean neural networks capable of analyzing more than one data type. Mainly, they focus on audio-visual data: an example of which you can find in this Microsoft paper.
Transformers have been traditionally used in NLP and are founding blocks of many SOTA architectures for text classification, sequence labelling or question answering.
However, since the introduction of Vision Transformer and TabTransformer in 2020, transformers have been successfully applied to computer vision and tabular data outperforming CNN and GradientBoosing approaches. The challenge in using transformers to images and tables lies in the lack of established self-supervised pre-training approaches present in NLP in the form of language modeling.
I believe 2022 will be fruitful in new transformer-based models bridging the gap further between different modalities with the focus on finding self-supervised pre-training objectives.
RGB imaging is the most widely-known field explored by AI researchers. We see a lot of problems solved using RGB-imaging and deep learning models. But recent advancements in hardware and data processing power have led to machine learning being used with much more complex data types. A technology that is likely to gain popularity among AI researchers in 2022 is Hyperspectral Imaging, which, in contrast to RGB-imaging, can provide images with more than three channels.
A perfect example of a similar trend is how deep learning models are now used to segment the data caught by complex data capturing devices, including LiDAR (Light Detection and Ranging) sensors.
LiDAR uses a laser pulse to collect measurements and create 3D maps of an environment, which — when combined with AI — helps autonomous vehicles tell the difference between humans and trees.
In my opinion, the most promising but still overlooked vision technology is Hyperspectral Imaging (HSI). Specialized HSI cameras can operate in the near-infrared wavelength range of light. Thanks to this, they can capture information about chemical and biological characteristics, opening up completely new use cases, such as early skin disease detection and non-contact food safety and quality inspection.
In the next few years, I believe we will see much higher adoption of Hyperspectral Imaging (HSI) in different industries and a massive increase in machine learning techniques dedicated to solving problems using Hyperspectral Imaging.
Medicine and healthcare are slowly embracing AI. And thanks to new infrastructure and technologies, the quality of medical services is getting better by the day. Artificial intelligence isn’t just able to diagnose patients quicker. It can help physicians offer more personalized treatment for everyone.
The WHO estimates that demand for healthcare employees will rise to 18.2 million across Europe by 2030, and the current supply will not meet current or projected future needs. The solution to this challenge could be AI. McKinsey estimates that 15% of current work hours in healthcare will be freed up by automation by 2030.
I believe the development of HealthTech AI is all ahead of us. Nowadays, we see a doctor on average once a year, and then we do a check-up and possibly a general check-up.
In contrast, we check and update our phones and computers once a month. In 365 days, our health can change dramatically, even developing cancer overnight. More data and more devices allow us to monitor our health daily.
I hope that AI in the health industry will move forward, and 2022 will bring us much-needed developments in this area.
As you can see, this year could bring big changes to the world of artificial intelligence. If the trends play out as we expect, they’ll alter how we live our lives and do business.
We want to thank the specialists for sharing their thoughts and giving us some insight into what to expect from the year ahead. If you want to stay up to speed with the wonderful world of AI in 2022, here are two great options:
That’s all for now, but we’ve got plenty of big projects of our own in 2022, so stay tuned for updates.