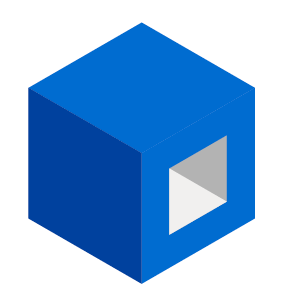
Biggest Strengths
Find out the core characteristics that make each Machine Learning framework so popular
Check out what to learn in 2021, and use technology that brands like Google, Airbnb, Spotify, Coca Cola trusted.
see what it takes to build your own AI-powered object detection software using deep learning and synthetic data, for things like:
Find out the core characteristics that make each Machine Learning framework so popular
See how companies use ML in eCommerce, Finance, FMCG, Travel, and more
Understand when a framework isn’t right for you, and which one to try instead
See what it takes to build your own AI-powered object detection....
Artificial intelligence is a $23bn market. And according to the latest estimates, it could pass $125bn by 2025. That’s a CAGR of over 30%, which is phenomenal by any standards. But growth alone isn’t a reason for an enterprise to deploy AI.
So today, we’ll answer the questions, “Why should forward-thinking leaders, like yourself, explore ways to use AI or ML in your organization? And should you even care about the top ML frameworks in 2021?”
In short, we believe so: because artificial intelligence and machine learning can solve even the most complex business challenges.
To elaborate, the two technologies:
That said, the technology is still relatively young. Yet executives have increasing confidence in its power. Why so? Because industries from retail to BFSI, education to sports, marketing to finance, have piloted AI and yielded unprecedented results.
For a pertinent example: look no further than the turbocharged research and development of COVID-19 vaccines.
———
In the round, AI-ML is such a useful corporate tool because it unlocks agility in organizational DNA. Put another way: it improves operational effectiveness. It can even unlock new growth channels.
And the insights we’ll present today will show you how to leverage the right machine learning frameworks to accelerate your organizational growth. If you’d like first to step back and learn the differences between AI, ML, and data science, click here.
Once you feel up-to-speed, read on for the all-important details.
At DLabs.AI, we cash in on machine intelligence and efficiency to build scalable enterprise solutions. That means we know how to leverage technologies to solve complex business problems.
But what if your business doesn’t have this type of in-house expertise? That’s where off-the-shelf ML frameworks come in. But their availability benefits both experienced and less experienced operators for three reasons.
Machine learning frameworks mean you can:
ML frameworks are potent resources. They allow an organization to reduce the developer resource required to complete a project. At the same time, they significantly shorten the time to deployment.
Here are the 10 frameworks to try in 2021.
With 152k+ stars and 83k+ forks on GitHub, Tensorflow is the open-source powerhouse of machine learning.
The Google Brain Team developed TensorFlow as a general end-to-end ML platform, using C++, CUDA, and Python. There are endless use cases for the framework, but to give you an idea, here’s how we’ve used it:
The language has a steep learning curve, so it isn’t ideal for beginners. TF is best deployed in multi-GPU environments but lags in performance in single GPU environments.
With 45k+ stars on Github, Facebook’s open-source ML framework, PyTorch, is a strong TensorFlow rival (it can do pretty much everything TF does). PyTorch is a community-driven Python package that’s intuitive, easy-to-use, lean, and fast — empowering developers to:
The primary differentiator of PyTorch versus other machine learning frameworks is its “reverse-mode auto-differentiation” technique, as illustrated below:
Traditionally, once neural networks are built, any modification requirements would translate into starting from scratch. But with reverse-mode auto-differentiation, PyTorch enables you to modify how your neural network behaves with almost zero overhead.
This means more flexibility and quicker development.
If we pit PyTorch against TensorFlow, we’d say TensorFlow is used comparatively more in deployment. But PyTorch has started to outpace TensorFlow in terms of research paper count.
Could this be a signal of PyTorch’s future share in ML model deployments? We believe so.
This isn’t necessarily a time to avoid PyTorch but, if you can, it’s often better to use TensorFlow Lite in place of PyTorch mobile for imparting ML capabilities on edge devices. Or, if you’re a JS developer looking for a robust ML framework, then TensorFlowJS could be a better option.
With 43.7k+ stars on GitHub, SciKit Learn is the third most popular framework for machine learning. It’s Pythonic (built on SciPy, Numpy, Matplotlib) and distributed under the BSD license.
SciKit Learn doesn’t suit GPU-based machine learning requirements. It’s also not built to run across clusters (Spark ML is more suited to cluster-based ML applications).
If you want to make predictions using data from a user’s device, that’s precisely what Apple’s core ML model lets you do. It integrates unified ML models directly into an app, using end-user data to make predictions, train the model — or improve the efficacy of the algorithm:
If you need a platform-agnostic ML framework, then this one isn’t for you. Expanding to other platforms could be a cost-intensive deal. For a full-stack, cross-platform machine learning framework, consider PyTorch or TensorFlow.
H2O is a distributed, scalable, extensible in-memory platform with 5.3k+ stars on GitHub. H2O provides off-the-shelf ML algorithms, like GLM, XGBoost, Random forest, DNN, GAM, and k-means. And it’s easily extensible with Hadoop, Spark, and other algorithms.
H20 isn’t the best for parallel running of multiple models while LDA and GMMs clusterings are also unavailable. If performance is your priority, consider open-source ML frameworks, like SciKit Learn, PyTorch, or TensorFlow.
And if you prefer a strong community, consider one of the ML frameworks listed earlier in this document.
Apache MXNET is an open-source, lightweight machine learning framework developed in partnership between Intel, the Apache software foundation, and an open-source community.
With 19.2k+ GitHub stars, the framework enjoys vast community support.
If you need more off-the-shelf features, there are frameworks that ship ‘with batteries included.’ And consider using SciKit Learn or Keras for research and prototyping.
FastAI enjoys 20k+ GitHub stars. Its mission is to democratize deep learning through its free MOOCs (massive open online courses).
FastAI boasts high-level components for building quick ML model prototypes — alongside low-level component access for researchers to experiment and build models with innovative approaches.
Some claim it’s difficult to implement low-level functionalities using FastAI, so it should be preferred more for research and learning and less for production.
Apache MLlib is part of the Apache Spark project. Apache Spark is highly popular among big data engineers, earning 28k+ stars on GitHub.
Apache MLlib lags in evaluation and result representation as its API doesn’t support direct extraction of probabilities with multiclass (Top-k error). The confusion matrix isn’t well displayed either — while for smaller data-sets, we prefer SciKit Learn.
PyTorch-lightning is a lightweight PyTorch wrapper for high-performance AI research.
It scores 11k+ stars on Github — and was designed for developing self-contained deep learning systems with highly-fragmented code to ensure maximum flexibility and minimum boilerplate code.
It helps to remember that Pytorch-lightning is just a high-level wrapper for PyTorch. If your organization has Pytorch talent, it makes sense to use it. — if not, look elsewhere. For smaller datasets, consider other solutions such as Keras.
CNTK is a robust framework with community-driven popularity of 17k+ stars on GitHub. But be wary that this unified toolkit doesn’t support model deployment to mobile devices.
The last stable release of CNTK was 19 months ago, highlighting the framework’s not-so-frequent updates. It’s also not ideal for delivering ML capabilities to mobile devices.
In that instance, Keras, IBM Watson, Azure ML, Google AI, and TensorFlow are good alternatives.
We’re sure that trends will change dynamically, so what’s top of mind this year may lose popularity the next.
If you want to read more interesting information from the world of Machine Learning — visit our blog.